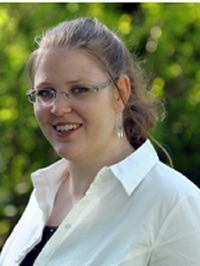
Calculating and Predicting Mood Changes in Cognitive Demanding Tasks Based on an Air Traffic Control Scenario by Martina Truschzinski
Abstract:
The focus of the presentation is the emotional and cognitive model we developed based on experimental data to investigate and compute changes in workload and mood during an air traffic controller task. The complete model consists of two separate models for workload and mood. The workload model is based on objective and continuously measured data which allowed us to develop a dynamic model to predict task-evoked pupillary responses. The model to predict mood changes is based on subjective data gathered via questionnaires. We analyzed the influences of the mood, which we measured by three dimensions good/bad, calm/nervous and tired/awake. Regression analysis showed that the mood is influenced by performance, workload, and personality, namely neuroticism and conscientiousness. Additionally, we found a strong dependency in mood and workload between their values before and after the task. The combination of both, the workload and mood model, is able to predict mood changes exclusively based on personality, mood before the experiment and the events happening during the air controller scenario.
Bio:
Martina Truschzinski studied applied computer science with the main focus on neuroscience at the University of Technology in Chemnitz, Germany. During her time as PhD student at the same university, Martina Truschzinski participated at two research projects: “The Smart Virtual Worker”, which developed an emotional and physical stress based autonomous agent model within different working contexts, and “MaCeLot”, which developed an emotional and cognitive model for cognitive demanding tasks. Thus, identifying the fundamental mathematical principles that underlie human thinking and behavior, and emotions, in particular, has been the main focus of her research since 2010.