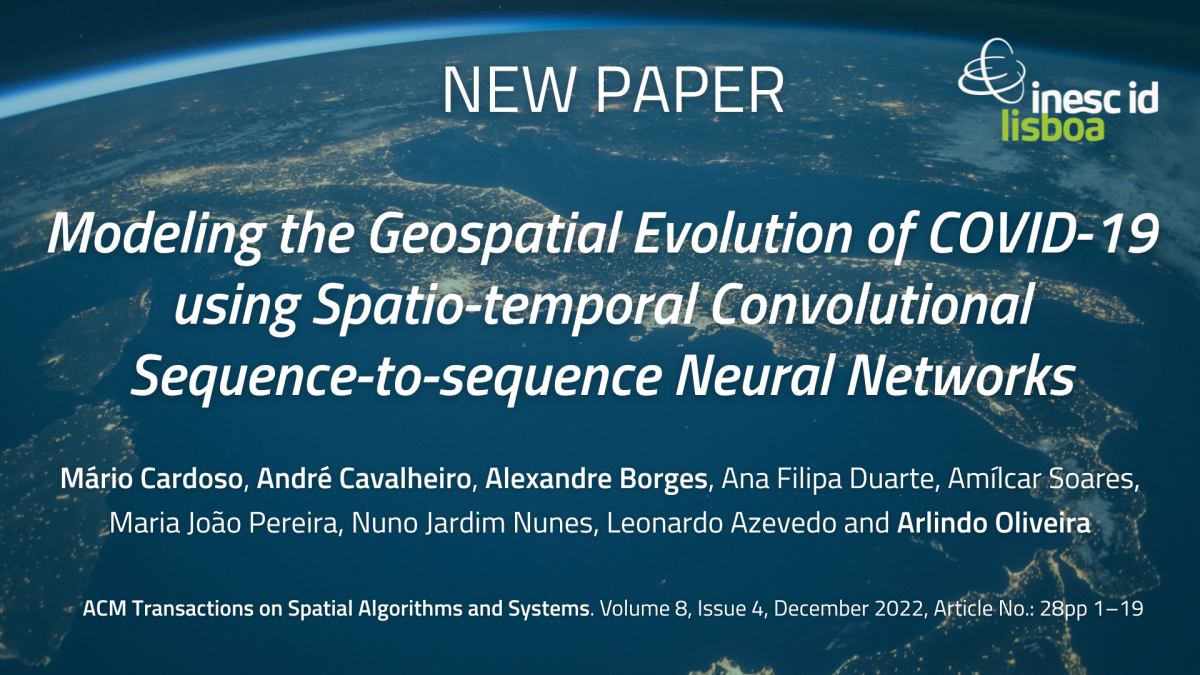
How and when viruses move: predicting the spread of COVID-19 using neural network models
A group of researchers, led by Arlindo Oliveira, has proposed a new method to compute the spatio-temporal dynamics of COVID-19 infections.
In a paper published this week on ACM Transactions on Spatial Algorithms and Systems, four INESC-ID researchers (Mário Cardoso, André Cavalheiro, Alexandre Borges and Arlindo Oliveira), together with colleagues from CERENA and ITI, implemented a neural network model — a computer program that simulates complex processes based on a very simplified form of how the human brain processes information — to estimate the incidence rate of COVID-19 in mainland Portugal.
Based on the STConvS2S (Spatiotemporal Convolutional Sequence-to-Sequence) Network architecture, and using data from the Portuguese Directorate-General for Health (DGS) across the first twelve months of the pandemic, these researchers were able to show that this type of network was the best performing method for mapping the geospatial evolution of COVID-19 (when compared to an Autoregressive Moving Average [ARMA] model, a Vector Autoregressive [VAR] model, and a Susceptible–Infected–Recovered–Dead [SIRD] model). In short, the best way to map the dynamics of this contagion between neighboring regions — an important step in making predictions about the evolution of pandemics.
All the data used in this article, as well as the code for the SIRD and the STConvS2S models, is available here.